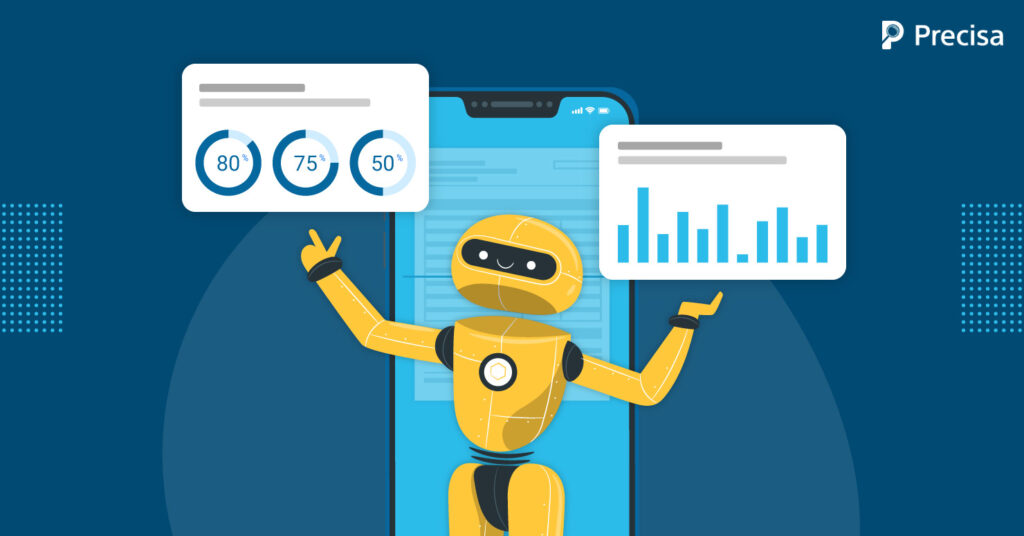
Whether it’s a bank lending to someone or a company giving credit to another, it all comes down to the borrower’s creditworthiness. Without evaluating this, losses are inevitable.
In the scenario of a bank lending money to people and companies extending credit to other companies, artificial intelligence (AI) has proven itself to be a powerful tool in this domain.
According to Allied Industry Research, AI in banking was valued at USD 3.88 billion last year alone. And by 2030, it’s expected to rise up to double-take-inducing USD 64.03 billion. Statista predicts that the AI market will rise twentyfold by 2030 and reach an astounding value of USD 2 trillion.
The future holds countless possibilities for AI when it comes to assessing credit risk. This article explores that potential along with the benefits and challenges that come with using AI in credit risk assessment.
What is AI for Credit Risk Assessment?
To evaluate credit risk, you must determine how likely it is that the borrower will fail to pay their financial obligations. There are traditional ways to do this, like looking at past data, credit scores, and financial documents.
While these practices have worked, there are a few issues. For one, they rely on data that is static. And two, they cannot adapt to changing economic conditions.
Artificial intelligence (AI) for credit risk assessment refers to technology such as machine learning algorithms that can predict if someone will be able to meet their payments or not. More intelligent risk assessments can be made by having access to huge datasets and alternative data sources.
The speed and accuracy of evaluating credit is improved with AI. This allows lenders to make faster and more informed decisions on whether or not they should lend money while avoiding human bias.
Understanding the Role of AI for Credit Risk Assessment
Credit risk assessment is being transformed in a number of ways by AI systems:
1. Predictive Analytics
AI systems can sift through large amounts of data and find patterns that we wouldn’t be able to spot. By looking at a borrower’s data, such as payment histories, income levels, and spending patterns, AI models can estimate their creditworthiness more precisely.
2. Risk Segmentation
Based on profiles, AI can categorise borrowers into multiple risk groups. This will allow lenders to give customised loan terms and interest rates, which will lower the risk of defaults and increase profit.
3. Real-Time Monitoring
Technologies powered by AI continuously keep an eye on borrower’s financial activity. In the event that a borrower’s financial condition worsens, it will send alerts to lenders so they are able to mitigate potential losses with proactive measures.
4. Credit Scoring and Machine Learning
Assessing credit risk heavily leans on machine learning, a variation of artificial intelligence. Models created by machine learning algorithms are more dynamic and accurate than conventional credit scores for a number of reasons.
They take into account more variables while adapting to changing economic conditions. Machine learning is also able to detect relationships in data that aren’t linear, increasing the accuracy of predictions made by it.
Advantages of AI for Credit Risk Assessment
The use of AI for credit risk assessment gives the following benefits:
1. Personal and Automated Decisions Throughout the Consumer Experience
Throughout a customer’s journey, AI models make several decisions for them. This automation is used to improve customer acquisition, relationship development, and smart servicing. As a result, the consumer experience becomes enhanced overall.
2. Making Credit Decisions Better
AI has a positive effect on the turnaround time and approval rates for credit approvals, enabling cost- and business-scalability reduction. More precisely, identifying riskier customers raises approval rates while lowering credit risk.
3. Detecting and Predicting Negligence and Fraud
AI assists in preventing fraud by instantly identifying suspicious activity. It is an essential component of credit risk management in the digital age since it lowers the likelihood of financial losses and legal action.
4. Optimisation of the Collections Process
AI algorithms use customer lifecycle data to determine which customers are most likely to repay loans. If red flags appear, institutions can take proactive measures to prevent defaults, saving money on third-party collections.
Challenges in AI for Credit Risk Assessment
Despite the potential of AI for credit risk assessment, there are challenges and factors to take into account:
1. Data Security
Utilising alternate data sources poses issues with data security and privacy, necessitating careful handling of sensitive data.
2. Model Interpretability
AI models can be complex, making it challenging to explain their decisions to regulators and borrowers. Transparency must be maintained.
3. Fairness and Prejudice
When improperly trained and monitored, AI models have the potential to unintentionally reinforce bias. Efforts must be made to ensure fairness in lending decisions.
4. Regulatory Compliance:
The use of AI in lending is subject to regulatory oversight. To stay compliant, lenders must manage constantly evolving regulations.
5. Governance
Sometimes, AI algorithms behave as opaque “black boxes,” making it difficult to understand how they make decisions. To meet this issue, business and technical teams must work together effectively and with proper governance, openness, and communication.
6. Quality
Data quality is crucial since quality AI depends on it. Poor decisions can be made as a result of inaccurate or unreliable data. Ensuring data quality is a constant challenge in AI adoption.
Future of AI in Credit Risk Assessment
The future of AI holds immense promise and potential in the realm of credit risk assessment. AI-driven innovations are set to revolutionize the way financial institutions evaluate and manage credit risk.
1. Advanced Data Analysis in Credit Risk Assessment
AI-powered enhanced data analysis will open up new perspectives for credit risk assessment in the future. By including unstructured data from various sources in addition to structured data, it allows for a more comprehensive assessment of borrowers’ creditworthiness.
2. Better Predictive Models for Risk Assessment
In terms of credit risk assessment, the future holds increasingly reliable and precise forecasting models. Deep learning and reinforcement learning, two types of AI, will be used to find complex patterns within enormous datasets, improving risk prediction and lending decisions.
3. Risk Monitoring and Mitigation in Real-Time
Real-time risk monitoring will be a key component of AI-driven credit risk assessment in the future. Lenders may proactively identify and manage developing risks via AI technologies that continuously analyse borrower behaviour, transactions, and market dynamics.
4. Transparent Credit Decisions with Explainable AI (XAI)
It is crucial that credit decisions are transparent. In order to help institutions meet regulatory standards and foster confidence, explainable AI (XAI), which ensures AI models provide clear explanations for their credit evaluations, will become a focus.
5. Fair Lending with Customised Credit Scoring
AI will make it possible for credit scoring systems to be highly personalised and adapt to the needs of specific borrowers. This customization promotes fair lending practices, especially for those with unconventional credit histories or limited records.
6. Compliance and Ethics
Financial institutions will navigate regulations and prioritise ethical AI. Collaboration with legal experts and AI engineers ensures compliance, transparency, fairness, and bias prevention, promoting responsible lending.
The Bottom Line
AI is not just a technology for the future; it has been evolving for decades. For financial institutions, this is the ideal time to realise their worldwide potential.
The adoption of AI for credit risk management has only begun, and the potential applications go far beyond the world of banking. Early adopters of AI have a competitive advantage in the ever-changing banking and other industries.
Precisa’s Bank Statement Analyser is an effective tool for classifying, verifying, and analysing bank statements to uncover abnormalities or manipulations. Our analyser produces the most accurate results, improving your credit appraisal quality.