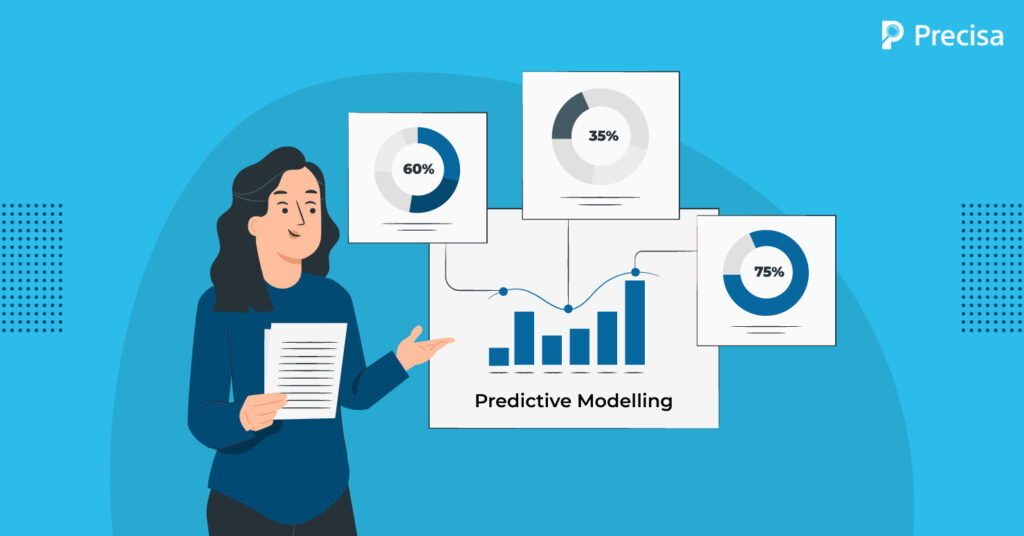
A balance sheet is a vital financial statement that summarises a company’s assets, liabilities, and equity status as of the reporting date. Data in a balance sheet serves as the foundation for determining the firm’s financial performance, the resilience of its fundamentals, and forecasting its future performance. The principal use of balance-sheet analysis is for credit appraisal and risk assessment in the context of lending or investing.
With the introduction of new technologies such as machine learning (ML) and data mining, many financial statement analysis software emerged, assisting fintech companies in simplifying their operations and scaling their businesses.
The use of predictive models is an emerging practice in financial analysis. In the year 2020, the global market for predictive analytics software was $5.29 billion. Experts predict that it will reach $41.52 billion by 2028.
This article explores the application of predictive models for balance sheet analysis to improve the efficiency and accuracy of credit appraisal processes.
Why is Predictive Modelling Necessary in Balance-Sheet Analysis for Credit Appraisal?
McKinsey Global Institute’s global balance sheet, a new line of research for evaluating the wealth and strength of the global economy developed in 2021, emphasises the relevance of balance sheet analysis in accounting and finance.
However, while balance sheets provide valuable financial information, they have an inherent limitation- the costs recorded are historical.
Nicolas Darvas, a renowned self-taught investor and the originator of the widely used Darvas Box Theory for stock trading, once said:
“All a company report and balance sheet can tell you is the past and the present. They cannot tell future.”
Credit analysts understand the reality of this remark better than anyone else. To obtain a more comprehensive view of a firm while performing balance sheet analysis for credit appraisals, they work with projected and estimated balance sheets in addition to actual figures for the current and prior years.
The projections and estimates are prepared in good faith by certified financial analysts. However, they frequently lack accuracy since they are based on judgements or even sometimes are purposefully adjusted to reflect a stronger financial situation than reality.
In this context, innovative processes like predictive modelling use cutting-edge technologies to mitigate the shortcomings of manual processes and improve the quality of credit appraisals.
Predictive Analytics and Predictive Modelling for Credit Appraisal: Understanding Subtle Differences and Convergences
While the terms predictive analytics and predictive modelling are sometimes used interchangeably, there is a distinct difference between the two.
1. Predictive Modelling
Predictive modelling use statistical approaches to evaluate existing data and extrapolate future outcomes, events, or behaviour based on this analysis.
Algorithms are used iteratively in predictive modelling to ‘train’ models to forecast the most accurate future scenario. Typically, ML algorithms are used in predictive modelling.
2. Predictive Analytics
Predictive analytics is a broader realm that employs inferences from predictive modelling to solve business problems.
For instance, consider the case of an e-commerce site. Based on prior years’ data, it can utilise predictive modelling to forecast the volume of sales expected in a forthcoming Black Friday sale.
Predictive analytics, on the other hand, will assist the seller in understanding which customer segments are most likely to respond to a specific sales offer.
Predictive modelling is more of a technique used or an aspect of predictive analytics. To put it simply, predictive analytics is an analytical technique that employs predictive modelling as one of its tools.
An Overview of Predictive Modelling Techniques
Predictive modelling techniques fall into two classes, namely parametric and non-parametric. Some of the most widely used predictive modelling techniques are:
1. Logistic regression and linear regression
Regression analysis estimates the outcome of events based on the correlation between dependent and independent variables in a dataset.
2. Neural networks
Also called Artificial Neural Networks(ANN), these models mimic human brain functions. They can review large volumes of complex data and identify abstract relationships in the dataset.
3. Time series algorithms
Time series algorithms estimate the outcome for a desired value based on historical data. They depict data in chronological order and can be used for predicting continuous values across time.
4. Decision trees
Decision trees follow the typical flowchart structure and are used to classify or forecast data based on how a set of questions is answered.
This model is notably beneficial for understanding an individual’s decision-making process and, as a result, for determining borrowers’ creditworthiness during appraisals.
5. Outlier detection algorithms
These models are used to find out anomalies in the properties and behaviour of data objects in a given cluster of data.
6. Ensemble Models
Ensemble models integrate the findings from multiple machine language algorithms to provide a more accurate forecast.
Predictive Modelling in Balance-Sheet Analysis for Credit Appraisal: 4 Major Benefits
Five key advantages of employing predictive modelling techniques in balance sheet analysis for credit appraisals are listed below.
1. More precision in forecasting future figures
Working with balance sheet data that are calculated or projected based on guesswork is difficult for credit analysts. Subpar data will affect the appraisal resulting in an eventual decline in asset quality.
For the line items in estimated/ projected balance sheets, predictive modelling techniques can provide more accurate figures based on past data.
2. Better credit risk analysis
Balance sheet components aid in determining the applicant’s liquidity, solvency, and overall credit risk. Modelling tools analyse data to discover patterns in default/delinquency percentages and forecast risk levels in future loans. In a sense, the model risk profiles the application.
3. Reduced costs and lesser turnaround time for processing
Balance sheet analysis, which used to take analysts hours to complete, is now completed in minutes thanks to predictive analytics and modelling tools. This minimises the time it takes to process loan applications. Less manual labour eliminates the need for dedicated personnel, thus lowering expenses.
4. Fraud prevention
Predictive tools and ML are equipped to detect fabricated and manipulated balance sheets, resulting in lesser instances of credit fraud.
Final Note: Points to Remember
Predictive modelling has its sure-shot benefits while doing balance-sheets analysis for credit appraisals. However, one should exercise caution when using such tools. These models rely on historical data for generating forecasts. Hence the accuracy of the output will depend on the authenticity and reliability of past data.
Analysts must also understand which predictive model to be used, how to implement it, and how well their data set is compatible with the chosen model. They must start by stating what they intend to achieve from the process and how the results will be used. The utility of predictive analytics is increased when there is a clear vision of the final goal.
Presica provides a cutting-edge bank statement analyser that can retrieve real-time transaction data via Account Aggregator. Over 1.25 lakh bank statements have been processed by our bank statement analyser. The tool cuts credit processing time by5 times. For further information, please mail to contactus@precisa.in.