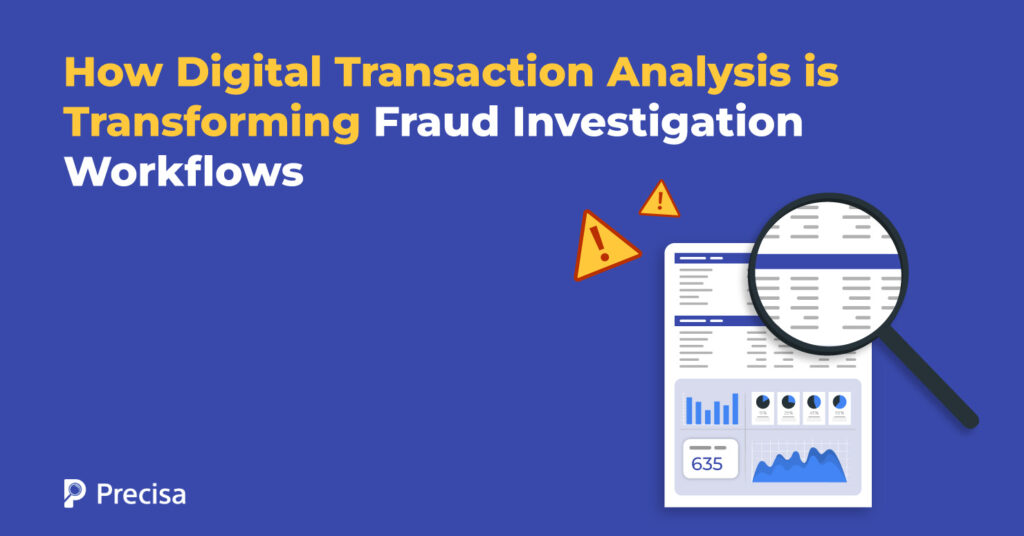
India’s digital transactions skyrocketed from 2,071 crore in FY 2017-18 to 18,737 crore in FY 2023-24, with a stunning CAGR of 44%. While digitisation brings convenience, it also fuels sophisticated fraud threats. However, the solution may lie within the problem – analysing digital transactions to combat this menace.
The growth of digital payment systems creates vast data caches that, if analysed effectively, provide valuable insights. The analysis reveals patterns and irregularities indicative of fraudulent activity. This is why digital transaction analysis can greatly help in fraud investigation and prevention.
Read on to find out how it can assist lenders in investigating fraud.
What Is Digital Transaction Analysis?
Digital transaction analysis involves evaluating electronic transaction data to identify patterns and detect anomalies. Leveraging data analytics, AI, and machine learning helps evaluate transactions across various channels to identify suspicious activity in real time.
For example, in partnership with Google, HSBC developed an AI system called Dynamic Risk Assessment, which enhances the detection of financial crimes. It analyses approximately 1.35 billion transactions monthly across 40 million customer accounts.
Key Components
- Real-time Monitoring: Lenders gain immediate insights into payment activities, facilitating prompt decision-making and proactive risk management.
- Data Visualisation: Transaction data is presented in insightful charts, graphs, and dashboards, enabling lenders to quickly identify patterns, anomalies, and trends for more informed lending decisions.
- Fraud Detection: AI algorithms analyse transaction data to identify suspicious patterns and flag potentially fraudulent activities, protecting lenders from financial losses.
- Personalisation: Transaction history analysis allows lenders to create personalised offers and tailor financial products to individual customer needs, fostering stronger customer relationships.
- Spending Pattern Analysis: Lenders gain a deeper understanding of customer spending habits, enabling the development of more effective budgeting tools, expense trackers, and financial planning services.
How Does Digital Transaction Analysis Aid Fraud Investigation?
Now, let us focus on how the transaction analysis helps with fraud investigation:
1. Pattern Recognition and Anomaly Identification
The system creates models of normal behaviour for each customer by analysing historical transaction data benefitting lenders in the following ways:
Machine Learning Powered Insights
Advanced algorithms analyse vast transaction volumes swiftly, detecting complex and evolving fraud patterns, such as abrupt spending spikes or frequent small purchases designed to evade alert thresholds.
For example, In 2019, JPMorgan Chase applied fraud analytics to reduce credit card fraud by over 30%. It used machine learning models trained on transaction data to predict fraudulent behaviour.
Flag Anomalies in Real Time
Data transaction analysis aids fraud investigation by automatically flagging unusual activity that deviates from established behaviour patterns.
Digital transaction analysis systems can generate immediate alerts, allowing the account holder or the organisation to act before matters escalate.
2. Dynamic Strategies for Fraud Investigation
A Business Standard report revealed that Indian citizens suffered losses exceeding Rs 1,750 crore due to cybercriminal activities between January and April 2024. Using the latest technology can deal with such growing challenges.
Advanced transaction monitoring tools that leverage artificial intelligence adapt and learn from new data. These systems dynamically adapt to emerging fraud patterns and modify their models and rules accordingly.
Predictive analysis models help identify the likelihood of fraud. As these models are constantly updated with new data, dynamic strategies can be formulated around these insights, allowing investigators to anticipate fraudulent activity before it happens.
3. Accurate Risk Assessment
Fraud investigation involves not only examining incidents of fraud after they occur but also the prevention and identification of potential fraud. Lenders can achieve this through the following approaches:
Develop Customer Profiles
Transaction analysis helps build customer profiles based on spending habits, preferred merchants, and transaction amounts. This helps create a baseline of “normal” conduct and deviations from the baselines, which can be flagged for further investigation.
Lenders can use the analysis insights to monitor their portfolio and lend to low-risk applicants.
Adopt a Focused Approach
Understanding individual customer behaviour helps the system reduce the number of false positives, allowing investigators to have a focused approach and target real threats.
4. Improved Efficiency
Lenders must be prompt in their fraud investigation to prevent further damage and ensure the fraud is not replicated. Data analysis improves efficiency in the following ways:
- By automating the identification of suspicious transactions, investigators can focus more on high-risk cases, reducing the overall investigation time.
- Automating data analysis and anomaly detection reduces the time required to sift through data and identify problems.
- It also improves accuracy in fraud detection as investigators spend less time investigating false alarms.
- Integrating tools like financial statement analysis into the lending process can reduce the time spent on data gathering and reconciliation.
5. Integration with Regulatory Databases
Digitisation has allowed different organisations to interact with each other, and data is no more confined to silos. Integration with regulatory databases offers the following advantages in fraud investigation:
Access to Watchlists
Integrating digital transaction analysis tools with regulatory watchlists and sanctions databases helps lenders monitor and identify transactions involving high-risk individuals.
Smoother Regulatory Compliance
Transaction data analysis tools assist financial institutions in adhering to anti-money laundering (AML) regulations and Know Your Customer (KYC) requirements by identifying and reporting suspicious activities.
The tools can generate suspicious activity reports, helping organisations meet regulatory reporting standards more efficiently and accurately.
In Conclusion
Digital scams are getting more complex, so lenders need to use the latest technology to protect themselves and their customers. Digital transaction analysis can be instrumental in organisations preventing fraud and investigating suspicious activity.
As a cloud-based analytics platform, Precisa offers the following solutions to assist FIs in fraud investigation and prevention:
- The Bank Statement Analyser automatically extracts data from the bank statement, classifies and categorises transactions, analyses the data, detects fraudulent transactions and other anomalies, and assigns an overall creditworthiness score.
- The GSTR Analyser verifies GSTIN, generates GST compliance ratings based on GST filing status, extracts data from the uploaded files, analyses the data and detects cyclical transactions and other anomalies.
- Credit Reports Analyser connects to Credit Bureau data sources and analyses the data, generating cross-analysis of Credit Report and Bank transactions data.
The tools have processed over 55 million transactions across 1000+ formats to help lenders deal with fraud, reduce risk and streamline operations.
Want to find out how we can help you in your operations?
Schedule a demo now.